Combined metabolomics and genomics approach for the diagnosis of inherited metabolic disorders (IMD)
Volume 16, Issue 2, July 2021 (download full article in pdf)
Editorial note:
In this topical update, Dr Eric Law reviews and updates on the current development in metabolomics and genomics and their integrated approach in the study of inherited metabolic disorders (IMD). We welcome any feedback or suggestions. Please direct them to Dr. Sammy Chen of Education Committee, the Hong Kong College of Pathologists. Opinions expressed are those of the authors or named individuals, and are not necessarily those of the Hong Kong College of Pathologists.
Dr Chun-yiu LAW
Consultant, Division of Chemical Pathology, Department of Pathology, Queen Mary Hospital
Introduction
Inherited metabolic disorders (IMD) refer to a group of heterogeneous biochemical disorders involved in different pathways of metabolism in humans. Metabolism refers to biochemical processes that occur in cells. These are the fundamental chemical reactions related to cell viability, growth, division, etc. Metabolism can be broadly classified into two major processes. One is catabolism, the production of energy from various nutrients, such as glucose, fat, and amino acids. The other process is anabolism, the biosynthesis of new cellular components, such as protein synthesis. A very early description of IMD was detailed by Sir Archibald E. Garrod about alkaptonuria back in 1902, who proposed the conditions to be inheritable and was caused by a specific enzymatic defect [1]. Indeed, IMD is more than just an enzymopathy and the knowledge of IMD and the human metabolome is still expanding in the next 100 years after the discovery of alkaptonuria. A computational analysis of the complete human genome has assigned 2,709 human enzymes to 896 bioreactions [2]. A more recent annotation includes 3,044 human molecular pathways covering 9,022 gene products [3]. According to the latest human metabolome database (HMDB version 4.0), there are 115,398 metabolites that linked with 5,702 different proteins [4].
The human metabolomes
Knowledge of the human metabolomes and their metabolic interactions is important for the understanding of human diseases. For example, it is now recognized that mitochondria are not only a factory for oxidative phosphorylation and energy metabolism, mitochondria also orchestrated with over 1,000 proteins and linked with multiple biochemical processes [5]. Indeed, a disrupted mitochondrial homeostasis had also been observed in some organic acidurias [6, 7]. The interactions of human metabolomes are far more complex than once perceived and involve different cell types, diets, drugs, disease status, microorganisms, and many others (Figure 1). The collective ‘big picture’ can be better studied through exometabolomics [8-10]. For example, acetic acid and trimethylamine (TMA) have been identified as biomarkers of bacterial urinary tract infection (UTI) and Escherichia coli associated UTI, respectively [11, 12]. TMA is one of the examples of mammalian-microbial co-metabolism which the host metabolizes TMA into trimethylamine N-oxide (TMAO) via flavin monooxygenase 3 (FMO3), and in E-coli associated UTI, the endogenous TMAOs are converted back to TMA possibly through the action from bacterial TMAO reductase. Similar mammalian-microbial co-metabolism has been described in aspects of human health that include cardiovascular disease, immunity, gastrointestinal disorders, and cancer [13-16]. The whole metabolomics network is more complex when toxico-metabolomics from drugs, chemicals, and environmental pollutants are taken into account. To-date, 2,280 drug and drug metabolites have been reported in the DrugBank database, and 3,670 toxins and pollutants have been reported in the Toxin and Toxin Target Database [17, 18], and the databank is still expanding.
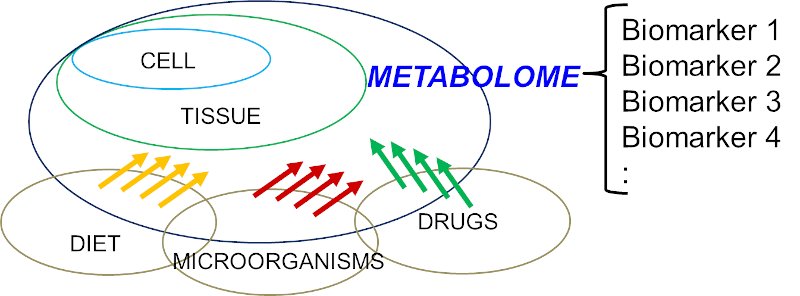
Figure 1: Simplified diagram to illustrate the structure of a human metabolome which is a complex interplays between host (human) and various factors, e.g. diet, microorganisms, drugs, etc.
Metabolism is the heart of many disease processes. Insights gained from the knowledge of metabolism will inform diagnoses and lead to new treatments. For example, 116 treatable intellectual disability caused by IMD has been described in a 2021 review [19]. In this newsletter, more emphasis will be put on IMD, a heterogeneous condition involving disorders of synthesis, catabolism/anabolism, transport, and storage of metabolites.
Classifications of IMD
The definition for IMD is further refined as described in [20]. Indeed, the presence of an abnormal metabolite is no longer essential for the classification of IMD, but instead includes any condition resulting in the dysfunction of the specific enzymes or biochemical pathways that is intrinsic to the pathomechanism. In individuals, IMD is rare. However, in a population they are collectively “common”. The estimated incidence of IMD is 1 per 4,122 to 4,355 live births [21, 22]. The true prevalence of IMD is difficult to measure due to various factors. It was estimated as 1 in 800 to 2,500 newborns in one study in 2020 [23]. According to the Society for the Study of Inborn Errors of Metabolism (SSIEM), there are over 600 different IMDs, and they are grouped into 15 hierarchical classifications based on the biochemical pathway involved. They are (1) disorders of amino acids and peptide metabolism, (2) disorders of carbohydrate metabolism, (3) disorders of fatty acid and ketone body metabolism, (4) disorders of energy metabolism, (5) disorders of the metabolism of purines, pyrimidines and nucleotides, (6) disorders of the metabolism of sterols, (7) disorders of porphyrin and haem metabolism, (8) disorders of lipid and lipoprotein metabolism, (9) congenital disorders of glycosylation and other disorders of protein modification, (10) lysosomal disorders, (11) peroxisomal disorders, (12) disorders of neurotransmitter metabolism, (13) disorders of the metabolism of vitamins and (non-protein) cofactors, (14) disorders of the metabolism of trace elements and metals, and (15) disorders of and variants in the metabolism of xenobiotics (For more details, please refer to https://www.ssiem.org/resources/resources/inborn-errors-classification). This is a 2012 classification from SSIEM. Knowledge of IMDs is still expanding with the advancements in next-generation sequencing (NGS) which has led to the discovery of more disease-causing genes and disease classes in IMD. For example, new class, such as congenital disorders of autophagy, which cause multiple system involvement have been described in patients with inborn errors of neuro-metabolism [24].
Examples to enhance diagnostic workflow in IMD
Recently, the International Classification of Inborn Metabolic Disorders assigned 1,450 monogenic conditions related to metabolism to 24 categories [25]. These conditions can have overlapping signs and symptoms. Some are rapidly fatal, mainly due to the accumulation of toxic metabolites and/or deprivation of energy; the diagnosis of IMD is clinically vital in this regard since it permits interventions to prevent further metabolic insults and irreversible damages. Unfortunately, there is no simple and single biochemical analysis that encompasses all pathognomonic markers in each IMD. Various methods had been described to decipher human metabolomes [26, 27]. Urine organic acid (UOA) via gas chromatography mass spectroscopy (GC-MS) was introduced in the 1960s and has since been adopted by most clinical laboratories. GC-MS is robust because it generates highly reproducible mass spectra, which allows positive identification using libraries, such as that of the National Institute of Standards and Technology (NIST). Nevertheless, there are several pitfalls of GC-MS. These include low-level metabolites, co-elution, age-dependent variation of metabolite levels, etc. Data interpretation by pathologists is a labour-intensive process. For this reason, an in-house automatic solution was established to address the above pitfalls and assist the UOA reporting process. A checklist composed of almost 100 key metabolites was constructed using over 1,600 sets of UOA GC-MS data and partitioned according to different age groups. Positive identification of metabolites was defined according to their retention times and electron ionisation spectra. The 95th percentile for each compound and in each age group was used as a cut-off to define abnormally high OAs, which would be flagged for in-depth review by chemical pathologists. This algorithm allows: (1) a graphical display of individual UOA levels and comparison with controls according to different age groups, (2) calculation of ratios of metabolites useful in interpreting low-level, but clinically significant, metabolites, (3) pathway analysis by a holistic correlation analysis of all studied OAs, and (4) continual database enrichment. An example of a case of aromatic L-amino acid decarboxylase (AADC) deficiency, a neurotransmitter disorder is shown in figure 2 where a significant increase of vanillactic acid (VLA) is identified. Despite this refinement, the analytical process is time consuming and remains a bottleneck for rapid diagnosis. To further streamline the analytical process for IMDs, we further explored the use of nuclear magnetic resonance (NMR) spectroscopy for IMD diagnosis as an alternative or complementary for GC-MS analysis. This approach for IMD was proposed decades ago in 1999 [28]. To-date, at least 100 IMD conditions can be diagnosed by NMR spectroscopy, as reviewed by Engelke [29] and Moolenaar [30]. In addition, this technique allows for the identification of novel IMDs. Examples include aminoacylase 1 deficiency in patients with metabolic brain diseases [31], beta-ureidopropionase deficiency in patients with movement disorders [32], defective polyol metabolism in patients with leukoencephalopathy [33], and dimethylglycine dehydrogenase in patients with muscle disorders [34].
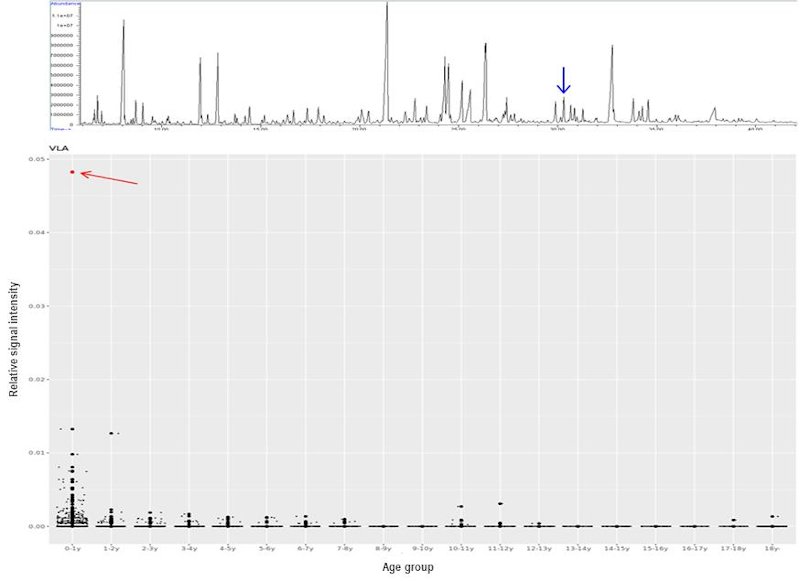
Figure 2: UOA spectrum by GC-MS from a case of aromatic L-amino acid decarboxylase (AADC) deficiency. The blue arrow points to the diagnostic marker, vanillactic acid (VLA) (Upper). Distribution of VLA levels in different age groups generated from in-house UOA algorithm. The AADC patient shows a marked increase of VLA (red arrow) comparing with age-matched subjects.
A national screening program in Turkey had applied NMR clinically to screen for IEM in newborns [35]. A total of 989 urine samples were collected from neonates and analysed by two laboratories. The results were used to establish a database and routine clinical screening. This NMR-based newborn urine screening has been further extended, covering up to 75 different IEM conditions [36]. The increasing awareness of clinical NMR applications has been further elaborated elsewhere in a 2021 review [37]. In our experience, the diagnostic utility of NMR has been substantiated in various clinical cases, for example, beta-ketothiolase deficiency, beta-ureidopropionase deficiency, citrin deficiency, fructose 1,6 bisphosphatase deficiency, holocarboxylase synthase deficiency, 3-hydroxyisobutyric aciduria, hyperornithinaemia-hyperammonaemia-homocitrullinuria (HHH) syndrome, methylmalonic aciduria (MMA), propionic acid, and succinic semialdehyde dehydrogenase deficiency (SSADHD). The merits of NMR-based urinalysis over GC-MS techniques are the simple sample preparation workflow and a relatively fast analytical time. Sample preparation is a two-step procedure that could be handled in
The choice of metabolic analysis depends on the nature of the pathognomonic metabolites. No single biochemical test that can detect them all. Pathologists have provided input concerning the choice of tests, advice on patient preparation and sample requirements, and clinical interpretations. The many examples include plasma acylcarnitine analysis, plasma/urine/CSF amino acids, urine acylglycines, bile acids, biotinidase activity, chitotriosidase activity, dried blood spot metabolic screening, homocysteine, transferrin isoelectric focusing, glycosaminoglycans analysis, CSF neurotransmitters, urine organic acids, urine sugars, urine guanidinoacetate and creatine analysis, urine oligosaccharides, orotic acids, red blood cells plasmalogens, porphyrins, phytosterols, pristanic and phytanic acids, purine and pyrimidines, very long chain fatty acids, and many more tests. Unfortunately, not all IMD-related tests are available or can be performed in a single centre.
The use of NGS will be a solution which provides additional insight from a genetic dimension, in particular if a biochemical assay is not available or the diagnosis cannot be easily demystified by biochemical tests. Primary coenzyme Q10 deficiency is one of the examples [38]. Affected individuals usually presented with non-specific symptoms and biochemical findings. Indeed, we have reported three cases of COQ4-related mitochondriopathy and identified a hotspot pathogenic variant in this locality using a genomics approach [39]. A plasma COQ10 assay was later developed for this potentially under-recognized condition.
Some IMD conditions are caused by multiple genes, for example, glutaric aciduria type II, methylmalonic aciduria (MMA), maple syrup urine disease (MSUD), propionic academia, phytosterolemia, congenital lactic acidosis, etc. Instead of a conventional gene-after-gene analysis, advances in NGS could allow the detection of the underlying genetic defect through a gene panel approach which effectively saves the time and manpower from managing a huge number of PCR primer bank and gene-specific protocols, not to mention the time spent in their revisions, updates and accreditations.
The diagnostic yield of NGS-based diagnosis for IMD is variable. In one report, NGS diagnosed 59% of the cases with clear clinical and biochemical features and a diagnostic yield of 8% for patients with an unclear phenotype [40]. Another group achieved an overall diagnostic yield of 50% and up to 78% in cases with a clear phenotype [41]. It is technically difficult to compare diagnostic yields of different studies, for reasons that include the scope of the IMD panel used, clinical and analytical aspects that can differ between centres.
Conclusions
With the expanding knowledge of metabolome and genome, more novel metabolites and genes have been discovered. These discoveries are enriching the understanding of IMD. Genomic and metabolomic analyses should be complementary to each other in the study of IMD, particularly in cases with atypical genetic findings or when a particular biochemical assay is not yet available. With the advancement of pharmacological chaperoning, small molecules and gene therapies, etc., more treatment options with improved care will be available in near future. At the same time, there will be increasing role from Pathologists for clinical use of cross-omics approach for disease diagnosis, monitoring and prognostication, with a more accurate and individualized characterization of disease progress.
Acknowledgement
The author would like to thank the supervision from Prof. Ching-wan Lam, Department of Pathology, The University of Hong Kong for his supervision on the NMR-related works. The author thanks Dr Gary Wong and Dr Jacky Ling, Division of Chemical Pathology, Department of Pathology, Queen Mary Hospital for their works on the in-house automatic solution in UOA analysis.
Reference
- Mussap, M., M. Zaffanello, and V. Fanos, Metabolomics: a challenge for detecting and monitoring inborn errors of metabolism. Ann Transl Med, 2018. 6(17): p. 338.
- Romero, P., et al., Computational prediction of human metabolic pathways from the complete human genome. Genome Biol, 2005. 6(1): p. R2.
- Sorokin, M., et al., Algorithmic Annotation of Functional Roles for Components of 3,044 Human Molecular Pathways. Front Genet, 2021. 12: p. 617059.
- Wishart, D.S., et al., HMDB 4.0: the human metabolome database for 2018. Nucleic Acids Res, 2018. 46(D1): p. D608-D617.
- Marchi, S., et al., MitopatHs: a new logically-framed tool for visualizing multiple mitochondrial pathways. iScience, 2021. 24(4): p. 102324.
- Chen, Z., M. Berquez, and A. Luciani, Mitochondria, mitophagy, and metabolic disease: towards assembling the puzzle. Cell Stress, 2020. 4(6): p. 147-150.
- Dimitrov, B., et al., Organic acidurias: Major gaps, new challenges, and a yet unfulfilled promise. J Inherit Metab Dis, 2021. 44(1): p. 9-21.
- Knott, M.E., et al., Metabolic Footprinting of a Clear Cell Renal Cell Carcinoma in Vitro Model for Human Kidney Cancer Detection. J Proteome Res, 2018. 17(11): p. 3877-3888.
- Lima, A.R., et al., Discrimination between the human prostate normal and cancer cell exometabolome by GC-MS. Sci Rep, 2018. 8(1): p. 5539.
- Palama, T.L., et al., Identification of bacterial species by untargeted NMR spectroscopy of the exo-metabolome. Analyst, 2016. 141(15): p. 4558-61.
- Lam, C.W., et al., NMR-based metabolomic urinalysis: a rapid screening test for urinary tract infection. Clin Chim Acta, 2014. 436: p. 217-23.
- Lam, C.W., et al., Quantitative metabolomics of urine for rapid etiological diagnosis of urinary tract infection: evaluation of a microbial-mammalian co-metabolite as a diagnostic biomarker. Clin Chim Acta, 2015. 438: p. 24-8.
- Claus, S.P., Mammalian-microbial cometabolism of L-carnitine in the context of atherosclerosis. Cell Metab, 2014. 20(5): p. 699-700.
- Brown, J.M. and S.L. Hazen, Microbial modulation of cardiovascular disease. Nat Rev Microbiol, 2018. 16(3): p. 171-181.
- Lin, L. and J. Zhang, Role of intestinal microbiota and metabolites on gut homeostasis and human diseases. BMC Immunol, 2017. 18(1): p. 2.
- Roager, H.M. and T.R. Licht, Microbial tryptophan catabolites in health and disease. Nat Commun, 2018. 9(1): p. 3294.
- Wishart, D.S., et al., DrugBank: a comprehensive resource for in silico drug discovery and exploration. Nucleic Acids Res, 2006. 34(Database issue): p. D668-72.
- Wishart, D., et al., T3DB: the toxic exposome database. Nucleic Acids Res, 2015. 43(Database issue): p. D928-34.
- Hoytema van Konijnenburg, E.M.M., et al., Treatable inherited metabolic disorders causing intellectual disability: 2021 review and digital app. Orphanet J Rare Dis, 2021. 16(1): p. 170.
- Morava, E., et al., Quo vadis: the re-definition of "inborn metabolic diseases". J Inherit Metab Dis, 2015. 38(6): p. 1003-6.
- Chong, S.C., et al., Expanded newborn metabolic screening programme in Hong Kong: a three-year journey. Hong Kong Med J, 2017. 23(5): p. 489-96.
- Lee, H.C., et al., Analysis of inborn errors of metabolism: disease spectrum for expanded newborn screening in Hong Kong. Chin Med J (Engl), 2011. 124(7): p. 983-9.
- Schuck, P.F., G.C. Ferreira, and M.C. McKenna, Recent advances in the pathophysiology of inherited metabolic diseases. Int J Dev Neurosci, 2020. 80(1): p. 50-51.
- Ebrahimi-Fakhari, D., et al., Congenital disorders of autophagy: an emerging novel class of inborn errors of neuro-metabolism. Brain, 2016. 139(Pt 2): p. 317-37.
- Ferreira, C.R., et al., An international classification of inherited metabolic disorders (ICIMD). J Inherit Metab Dis, 2021. 44(1): p. 164-177.
- Roca, M., et al., Reviewing the metabolome coverage provided by LC-MS: Focus on sample preparation and chromatography-A tutorial. Anal Chim Acta, 2021. 1147: p. 38-55.
- Tebani, A., C. Afonso, and S. Bekri, Advances in metabolome information retrieval: turning chemistry into biology. Part I: analytical chemistry of the metabolome. J Inherit Metab Dis, 2018. 41(3): p. 379-391.
- Bamforth, F.J., et al., Diagnosis of inborn errors of metabolism using 1H NMR spectroscopic analysis of urine. J Inherit Metab Dis, 1999. 22(3): p. 297-301.
- Engelke, U., et al., Handbook of 1H-NMR spectroscopy in inborn errors of metabolism: body fluid NMR spectroscopy and in vivo MR spectroscopy: SPS Publications, 2007. 2007.
- Moolenaar, S.H., U.F. Engelke, and R.A. Wevers, Proton nuclear magnetic resonance spectroscopy of body fluids in the field of inborn errors of metabolism. Ann Clin Biochem, 2003. 40(Pt 1): p. 16-24.
- Engelke, U.F., et al., NMR spectroscopy of aminoacylase 1 deficiency, a novel inborn error of metabolism. NMR Biomed, 2008. 21(2): p. 138-47.
- Moolenaar, S.H., et al., beta-Ureidopropionase deficiency: a novel inborn error of metabolism discovered using NMR spectroscopy on urine. Magn Reson Med, 2001. 46(5): p. 1014-7.
- Moolenaar, S.H., et al., In vivo and in vitro NMR spectroscopy reveal a putative novel inborn error involving polyol metabolism. NMR Biomed, 2001. 14(3): p. 167-76.
- Moolenaar, S.H., et al., Defect in dimethylglycine dehydrogenase, a new inborn error of metabolism: NMR spectroscopy study. Clin Chem, 1999. 45(4): p. 459-64.
- Aygen, S., et al., NMR-Based Screening for Inborn Errors of Metabolism: Initial Results from a Study on Turkish Neonates. JIMD Rep, 2014. 16: p. 101-11.
- > Embade, N., et al., NMR-based newborn urine screening for optimized detection of inherited errors of metabolism. Sci Rep, 2019. 9(1): p. 13067.
- Speyer, C.B. and J.D. Baleja, Use of nuclear magnetic resonance spectroscopy in diagnosis of inborn errors of metabolism. Emerg Top Life Sci, 2021.
- Yubero, D. and R. Artuch, NGS for Metabolic Disease Diagnosis. EJIFCC, 2018. 29(3): p. 227-229.
- Ling, T.K., et al., Clinical whole-exome sequencing reveals a common pathogenic variant in patients with CoQ10 deficiency: An underdiagnosed cause of mitochondriopathy. Clin Chim Acta, 2019. 497: p. 88-94.
- Ghosh, A., et al., Diagnosing childhood-onset inborn errors of metabolism by next-generation sequencing. Arch Dis Child, 2017. 102(11): p. 1019-1029.
- Yubero, D., et al., Targeted Next Generation Sequencing in Patients with Inborn Errors of Metabolism. PLoS One, 2016. 11(5): p. e0156359.